Vaccine (Dis)Information on Twitter
Traian Marius Truta, Alina Campan, Shawn Huesman, Celine Wardrop, Alyssa Appelman
Online social networks (OSNs) are a primary way to spread and consume information. In most OSNs, users can post and disseminate anything, and this can lead to a proliferation of inaccurate information. Fake news articles are being created and distributed, and their availability has become mainstream.
In this project, our goal is to analyze the volume and spreading pattern of tweets that are pro- vs. anti-vaccine.
To collect tweets on a specific topic, Twitter provides an Application Programming Interface — Streaming API — that allows users to collect tweets in real time. Twitter Streaming API provides two access levels: Free and Decahose. The Free option that we used in this work allows users to collect a sample of all public tweets or to collect a set of tweets that contain a predefined set of keywords. We focus here on filtered collection. Our initial experiments showed that during the collection time window, all tweets that contain one of the provided keywords are collected as long as there are fewer than 50 tweets per second. For vaccine-related keywords, we did not reach this high generation rate.
By reviewing existing work on vaccine-related tweets, we realized that there is no standard regarding which keywords to use. In some papers, the collection keywords contain one or very few keywords (e.g., vaccine, immunization, etc.) while in others, the collection contains as many as 72 words (e.g., mmr, zika, sica, anti-vax, anti-vaxx, etc.). As a result, we decided to create a workflow to find a comprehensive list of highly relevant keywords; the goal was to create the best sample representing the diverse opinions of the public on vaccines. First, we collected tweets for 60 minutes using the filtering approach with 80 keywords (the union of all keywords used in previous vaccine-related work). Our 60-minute collection yielded 7,323 tweets, each containing one or more keywords from our keyword set. Second, four researchers analyzed the first 50 tweets containing each keyword, annotating them as relevant or irrelevant to the topic of vaccines. For keywords with less than 50 corresponding tweets, all tweets were annotated. Third, we retained in the final list of relevant keywords all keywords with at least 10 matching tweets, and at least a 40% relevancy rating; the relevancy rating of a keyword was computed as the ratio between the number of matching tweets that were annotated relevant by all four annotators, and the total number of matching tweets.
Next, we will conduct an experiment on the keywords that had less than 10 instances in the first collected sample to determine if they are relevant or not. Then, upon completion of a comprehensive list of the most relevant keywords, we will collect data and build a classification model to automatically assign tweets to one of four groups: pro-vaccines, anti-vaccines, neutral, or irrelevant. Finally, we will analyze the spreading patterns of tweets that are pro- and anti-vaccine. These results will have implications for future researchers and practitioners in several disciplines, including epidemiology, sociology, and social network analysis. 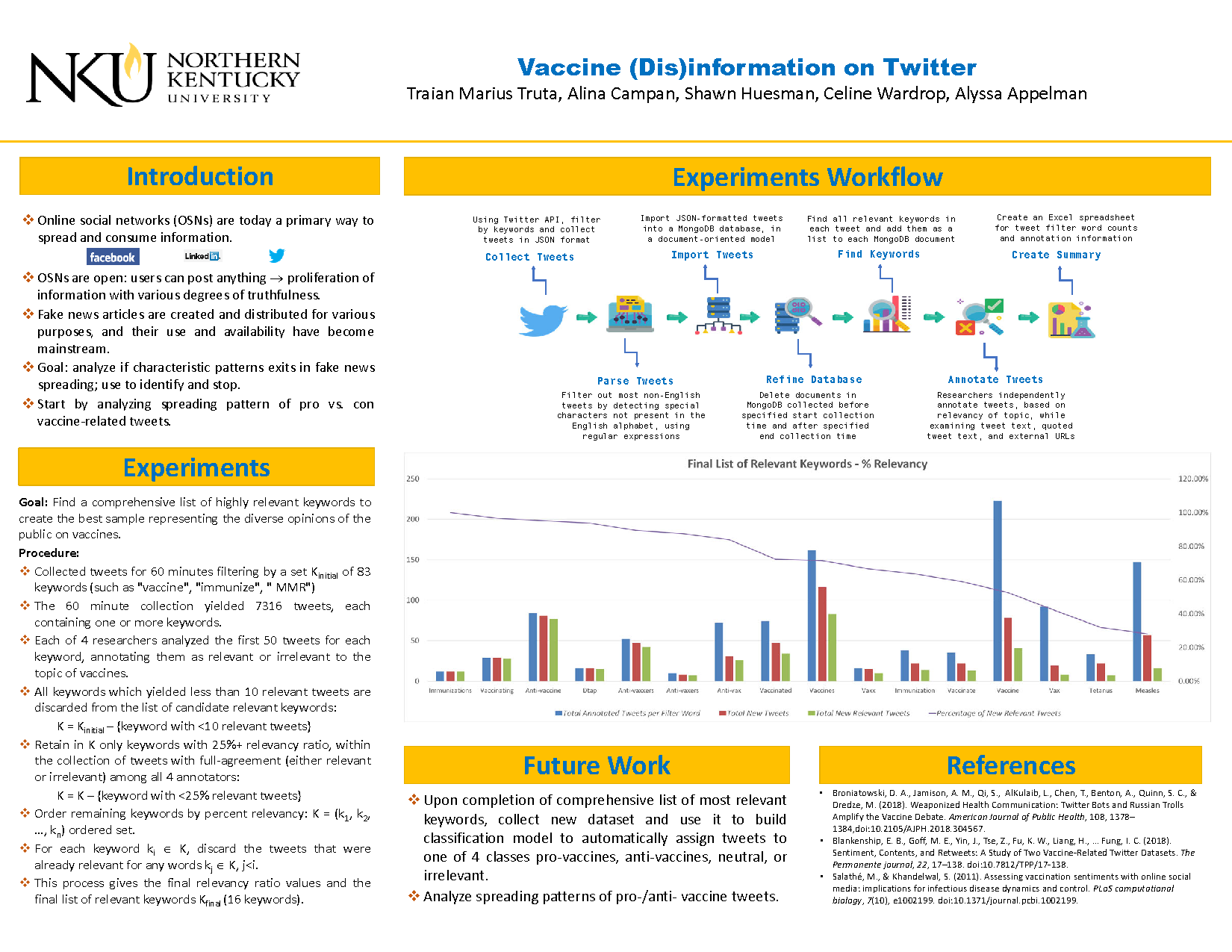
← Schedule